Most experts put the start of the Syrian Civil War on March 15, 2011, when, in the southern olive-growing city of Daraa, hundreds of people were killed and thousands arrested for protesting the torture of 15 students who had scrawled anti-government slogans on their school’s walls. But the conflict’s roots were far deeper and drier.
That March marked the fourth year of the worst drought the Fertile Crescent had seen since before Julius Caesar ruled the region. By the time Daraa had earned the moniker the “cradle of the revolution,” irrigation canals had run dry. Eighty-five percent of the country’s livestock lay dead in fallow fields; crop yields had fallen by two thirds; food prices had doubled. A million and a half Syrians had fled their homes for Aleppo and other cities already nearing breakpoints from the 1.2 million refugees who had poured into the country since the start of the Iraq War in 2003. Political fissures existed in Bashar al-Assad’s Syria before the drought. They became gulfs soon after the rains quit falling.
“The drought and the food shortages it prompted absolutely had something to do with the war that's still raging there now,” says Steve Price, a climate modeler at Los Alamos. It was a clear morning and Price, who is in his early fifties, was rocking back in his chair in his office, an array of solar panels and a fossil-fuel burning power station visible out the window behind him. Price was now working on a new modeling project that asked how, in an age when the global food supply must double over the next century to keep pace with population growth, the four crops—soy, wheat, corn, and rice—that feed five billion people every day will respond to the rapidly changing climate. Days earlier, he and Travis Pitts, a program manager in the Lab’s Global Security Directorate, had returned from a trip where they discussed whether their research could help the U.S. government and non-governmental organizations anticipate when a country might become prone to migration, conflict, or humanitarian crisis.
Syria, in many ways, offers a microcosm of what they were looking at. It is a place where human carrying capacity had been boosted by technology and then tested by an environment tilting toward extremes. Over the 70 years before the war’s outbreak, Syria’s population had gone from 4 million to 22 million. To feed the boom, the country’s farmers did the same things that the rest of the world did to feed a population that grew by 5 billion over that same timespan: They converted millions of acres of wildland to agricultural use. They boosted their crops’ productivity with new kinds of mass-produced fertilizer. And they built irrigation networks that connected the expanded farmlands to rivers watered by regular storms. Then, in a matter of a few short years, climate change erased generational gains. In October 2022, 12 years, 13 million displaced peoples, and 580,000 deaths into Syria’s ongoing civil war, Price and Pitts started a year-long modeling project to see if they could help answer a heavy question.
“Where,” he asks, “might Syria happen next?”
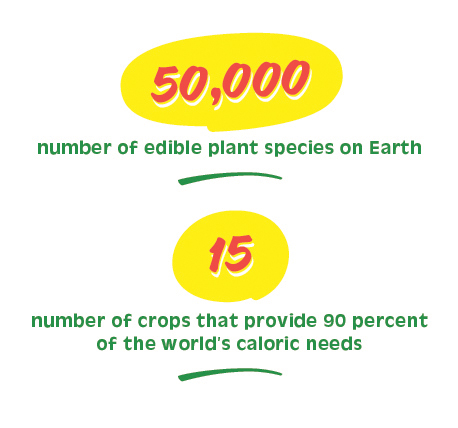
Food security is national security
In a 2021 report, the National Intelligence Council summed up the effects of climate change on national security as “increasingly exacerbating risks.” Harder but less frequent precipitation events will cause more soil runoff. Hurricanes, typhoons, wildfires, floods, droughts—all are projected to increase in frequency and intensity. At Los Alamos, scientists are coloring in the details of exactly how these expected changes will affect national security. Their efforts include high-resolution projections of U.S. naval bases being inundated by rising sea levels, forecasts on how melting sea ice may open new Arctic shipping lanes, estimates on how changes to precipitation and temperature may shift the habitats of disease vectors like mosquitos, and more. Regional instability caused by climate change is one area of research that the Lab is especially interested in, and food insecurity is one of the best predictors of political instability.
The National Intelligence Council says the effects of climate change are “increasingly exacerbating risks” to national security.
“My first publication,” Price says, pulling up on his computer a copy of a paper that he published in 1998. It was an analysis of ice velocities in one small corner of Antarctica. He and a dozen other scientists had built the dataset over a decade, collecting all the measurements by hand. “We’d fly to a location on the ice, take a measurement, fly to someplace else on the ice and do it again,” he says, pointing to a map of Antarctica on his wall. He spent five years working on field-based projects in Greenland and Antarctica before he came to Los Alamos in 2008 and his main focus switched to climate. Essentially a version of his 1998 paper, but at orders-of-magnitude higher spatial resolution, the color-coded map shows the speed that ice moved across all of Antarctica. “A satellite gathered the data to make this continent-wide map in a matter of months,” he says. It’s the same story for Earth system models. More data and better models are making it possible to answer questions that were unfathomably complex and labor intensive just decades ago. How climate change will affect food security is one of these questions.
Most Earth system models are built with many thousands of grid cells that are somewhere on the order of 100 kilometers by 100 kilometers by 1 kilometer deep. The depths of the atmosphere, the ocean, and the land are also broken into different vertical layers. Each cell is a three-dimensional column that is packed with equations representing hundreds of different factors specific to the cell’s area: precipitation, soil moisture, land and crop cover, the respiration rates of grassland versus deciduous forest, how the lifecycle of oceanic phytoplankton contributes to carbon cycling. By tying all of these factors together with neighboring grid cells and syncing them to time steps that move forward incrementally, Earth system models create a virtual representation of the global climate and all the processes affecting it.
Crop models are a relatively new addition to Earth system models. Traditionally, these empirical models, valuable mostly to economists and agriculture agencies, have operated as standalones that rely on relatively simple mathematics to consider how genetics, management practices, and the environment interact to determine crop yield. But crops, like all vegetation, are integral parts of Earth’s carbon cycle. It wasn’t until the past decade that supercomputers like those at Los Alamos, which run all manner of scientific models, became powerful enough for crop models to be included as standard components of Earth system models. The development allowed modelers to funnel some of the enormous amounts of data used to feed climate simulations into the relatively few bins that represent the bulk of the world’s food supply. This meant scientists could ask models pointed questions about the future of crops under different projected climate scenarios, and expect answers informed by a fair, if not quite comprehensive, representation of the processes that produce climate.
“That’s our innovative design,” says Chonggang Xu, a Los Alamos modeler who Price and Pitts asked to join the project because of his expertise in modeling vegetation under different climate scenarios. “Soil moisture, hydrology, canopy cover, energy balance—we now can leverage all of the Earth system models’ capabilities to answer questions in a way that wasn’t possible until very recently.”
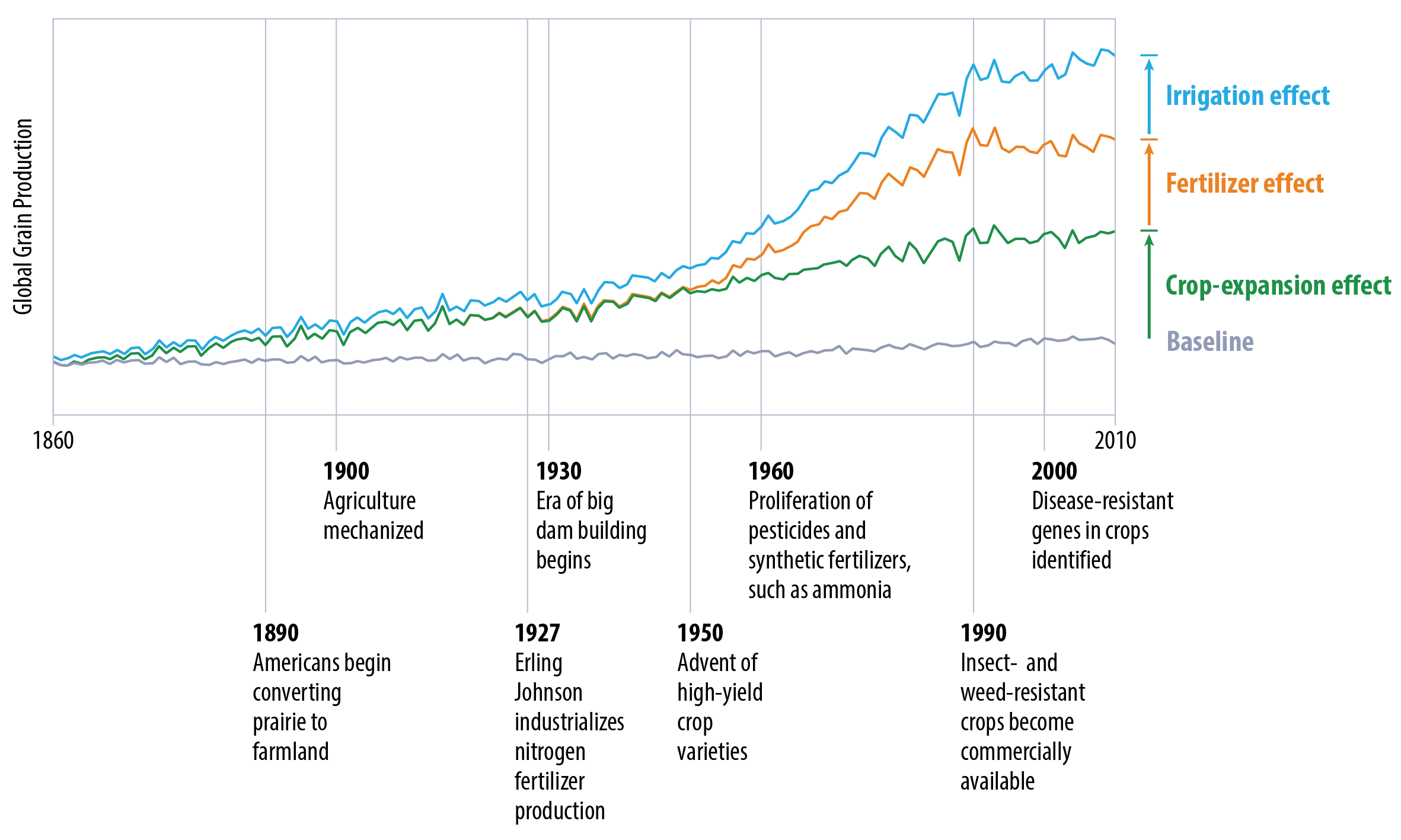
The setup
If chaos theory is right, and a chain of events that starts with a butterfly flapping its wings eventually causes a typhoon, the problem Earth poses to climate modelers is that the world is full of butterflies. Aberrations are everywhere. El Niño and La Niña; orographic and topographic effects; the circulations of ocean currents; re- and deforestation patterns. Taken together, the weave of connections that make up the climate system creates infinite future expressions of climate. As the International Panel of Climate Change (IPCC)—the United Nations body charged with assessing science related to climate change—puts it, modelers select a range of possibilities that includes some version of the likeliest climate outcome. “The goal,” the IPCC wrote in a recent report, “is not to predict the future, but to better understand uncertainties and alternative futures in order to consider how robust different decisions or options may be under a wide range of possible scenarios.”
To narrow the infinite down to that wide but representative range of future scenarios, climate modelers start every model run by picking any combination of two standardized sets of thousands of input variables. One set can be thought of as the model run’s final destination and the other as the road it takes to get there. The shared socioeconomic pathways, or SSPs, are the roads. The SSPs span five different narratives that represent the human choices that decide future climate: when and whether greenhouse gas emissions accelerate, decline, or remain steady; and the political, economic, technological, and social drivers behind those outcomes. The relative concentration pathways, or RCPs, can be thought of as the model run’s destination. These four distinct future histories are each defined by the percent difference in radiative forcing—the amount of energy, or heat, really, that enters the atmosphere compared to how much leaves it—between when the industrial revolution began in 1850 and the predetermined endpoint of the model run. That is typically 50 to 100 years out from the present. By employing a combination of SSPs and RCPs that range from mild to extreme change, modelers can compare versions of the future world and ask targeted questions of the results. If the U.S. transitions to renewable energies by 2030, will that translate to lower radiative forcing by 2045? And if there is lower radiative forcing in 2045, what does that mean for corn yields in Indonesia in 2060?
For their global food security experiment, which focused on the years 2015 to 2050, Price and his team selected a middle-of-the-road pathway: SSP 2 and RCP 4.5. Also known as SSP2-4.5, this combo assumes that, in terms of human choices, the past is a pretty good predictor of the future. Global, social, economic, and technological trends stay as they are now, with development proceeding unequally, environmental degradation continuing steadily, and the international consensus on how to stave off the worst of climate change wavering throughout the coming century. SSP2-4.5 predicts 2°C to 3°C of warming by 2100, a three-fold increase in extreme droughts, and a 35 percent rise in sea level compared to today’s levels.
To test how crop yields change under this scenario, Price and the team started their experiment by running the model from 1850, when humans started emitted greenhouse gasses in concentrations high enough to alter climate, to the modeling present, which is the year 2015 for the quirky reason that that’s when RCPs were first introduced. This method allows scientists to test the model’s fidelity by comparing the results to decades of real-world observations gathered by a network that now includes countless thousands of land-and-sea-based monitoring stations.
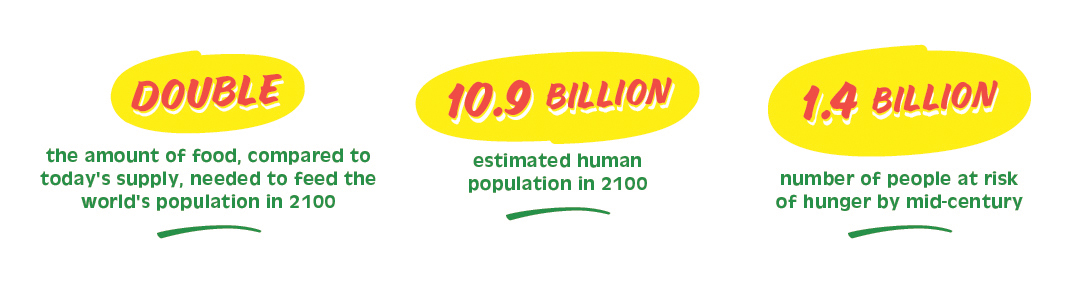
While the computers ran the model, Kurt Solander, a Lab hydrologist who is in charge of managing the team’s data, picked a few representative places around the world that would allow him to test the model’s forecasts against real-world observations. To compare how well the model captured crops’ responses to drought, he picked from the past 20 years a normal year and a drought year for both the midwestern U.S. and Western Europe. The data came from county harvest reports and satellite observations that projected crop productivity based on the amount of light reflecting off the crops. When Solander and Price compared the observational data to the model run, they found that the model had predicted yields that were slightly lower than those observed for both Europe and the Midwest. In neither case was this variance overly concerning because the model had captured the productivity drop associated with the drought, an important indication that the model was working. But then Price found something way off. In 2014, just before the historical run ended, the model’s global crop productivity dropped by a factor of four. Why?
Price dug into the data. The model had correctly forecast crop productivity climbing steeply from the 375 million tons of grain harvested globally in 1850 to the 2.4 billion tons of grain harvested in 2014. It captured the banner harvests associated with the mechanization of agriculture in the early 1900s. It showed the sky-shot trajectory continuing through 1927, when the Norwegian chemist Erling Johnson industrialized the process for creating nitrogen fertilizer; the productivity bumps linked to the onset of the big dam building era that began in the U.S. in the 1930s and continues globally today; the proliferation of pesticides and synthetic fertilizers—ammonia—in the 1960s; the clearing of the Amazon and the jump from 5 million to 80 million head of cattle that followed in the latter half of the last century. Not only had the model nailed the eightfold increase in global crop productivity, it had the drivers behind the trend correct. Yet there the predicted drop was. “It was weird,” Price says. And it remained so until he found the reason why: The model’s global crop yields plummeted by 75 percent because the world’s crops had gone unfertilized for a single growing season. In the model’s code, a typo had caused fertilizer use to expire a year early.
The discovery alerted Price to a disturbing possibility. Three primary factors led to the crop growth that powered last century’s global population boom: widespread fertilization and the expansions of both irrigation and cropland area. These variables are defined according to the socioeconomic factors that also determine greenhouse gas and aerosol emissions for a given scenario. Now Price wondered, what if that didn’t happen? What if all the best farmlands had already been planted? Or what if geopolitical hiccups or worse led to fertilizer shortages? The ongoing war in Ukraine hinted at an answer. Russia exports 13 percent of the world’s nitrogen fertilizer and provides nearly all of some countries’ supply. After Russia invaded Ukraine in 2022, sanctions and supply-line concerns caused global nitrogen fertilizer costs to jump from 200 dollars to more than 1200 dollars per metric ton. Shortly after, the number of people facing acute food insecurity reached a record high of 345 million, three times the number facing starvation just five years earlier.
Price fixed the fertilizer error in the model’s code and then added a new question for his project to answer. What would happen to crop yields if the climate changed but the things more immediately within human control—fertilizer use, irrigation use, and crop land area—were held at 2015 levels?
What would happen to crop yields if the climate changed but the world’s fertilizer use and crop land area were held at 2015 levels?
The results
Over the next eight months, the team ran the model many dozens of times using outputs from four of the world’s seven major Earth system models. “We sampled a lot of different potential future climates to get a handle on how big the uncertainty is in future crop yields,” says Price. Patterns slowly began to emerge. Price and his team chose as their metric the rate of year-on-year increase in crop production over historical and future-looking time periods: 1980–2010 versus 2020–2050. (The decade-long gap in the research was a product of modeling idiosyncracies).
At a glance, the story the models foretold looked mixed. Globally, over the next 30 years, wheat productivity is forecast to increase, with farmers pulling another 724 million tons of seed off the stalk each year. They will also harvest an additional 300 million tons of rice. But the models predicted a modest gain for soy, which will go from harvesting an additional 202 million tons per year to harvesting an additional 285 million tons per year. The future for corn, the world’s leading crop, is far worse. Productivity will actually drop from adding 899 million tons to adding 568 million tons per year. The findings grew more illuminating and nuanced when the team interrogated the results. How did the regional trends add up to a global story?
To get at this question, Solander wrote post-processing code that sifted through the mountains of data produced by four different Earth system models. Some conclusions rose to the top. Rising atmospheric CO2 actually fertilized wheat but didn’t fertilize corn. Wheat plants process CO2 in a way that allows them to take advantage of more atmospheric CO2, whereas corn plants process CO2 differently. That crop experienced essentially no productivity gains from the airborne fertilizer. Meanwhile, Xu used other codes to isolate the effects of droughts on crops. First, he ran the model and analyzed how the crops performed under more droughts. Then he ran the models under the exact same conditions but with the droughts removed. By comparing outputs from two runs that differed only by the presence or absence of droughts, the scientists were able to attribute how much the droughts, as opposed to other external factors, had contributed to future crop declines. Globally and on average, future drought impacts are forecast to reduce crop production by a modest ~2 percent. Regionally, however, the maximum annual impacts of drought are forecast to be much higher, with reductions of ~40–60 percent depending on the crop, region, and time period.
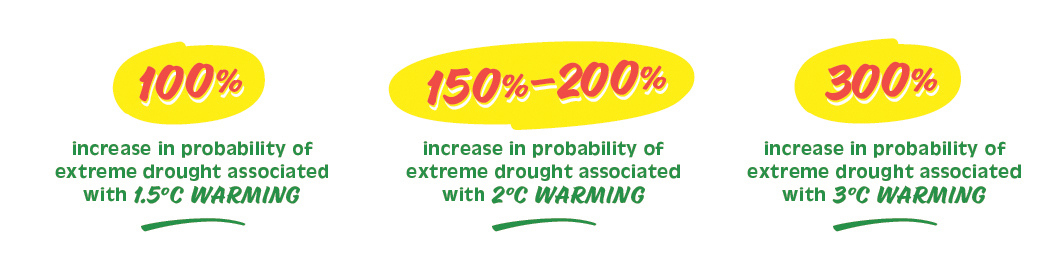
As Solander, Pitts, and Price continued to analyze the results, it became clear that the regional stories that explained the global trends were, well, messy. Take, for example, wheat. In the modeled future, the northern hemisphere is almost entirely responsible for the global wheat boom. Russia, where wheat yields fluctuated greatly between 1980 and 2015, will add more than 200 million tons of grain harvested per year. That story of gain held true throughout the mid-latitude northern hemisphere, places like Western Europe and the U.S., where the models predicted a near doubling of the increase in wheat harvests, from 365 million tons added each year to 603 million tons. The productivity bump is caused not only by CO2 fertilization, but also by the lengthening of growing seasons and the expansion of arable lands that is associated with the warming climate. These factors promoted such tremendous gains in the north that the parts of the world where productivity is forecast to drop failed to significantly pull down the global average. An example of shrinking harvests is South America’s mid-latitude countries, which includes Brazil. There, the model’s projected wheat productivity fell from 22 million tons of additional wheat grown each year to just 3 million additional tons grown each year.
Corn’s story is one of contraction. “For the most part, corn is in trouble,” says Price. The models predicted that lowland corn crops will not only fail to benefit from CO2 fertilization, but increasing temperatures will push them out of the now-too-hot river valleys where most corn is currently grown and into harder-to-farm higher elevations. In Latin America, which will grow by 100 million people over the next 30 years, corn productivity increases will drop by more than half, to just 23 million more tons harvested per year; the Middle East will lose 7 million tons harvested per year while doubling its population to 724 million. And Asia’s corn crop will go from adding 178 million tons harvested per year to adding just 63 million tons while the continent’s population climbs to 5.27 billion people—equal to the entire world’s population in 1990.
Adding to the complexity of these stories is that the models’ forecast shows monumental declines in corn productivity being somewhat offset by productivity boosts for other crops. In Latin America, rice yields are expected to increase by five million tons; in the Middle East, soy is going to pick up a million tons per year while rice gains five million. Will these gains compensate for corn’s loss? “It’s hard to extrapolate how this might play out in the real world,” says Price. “I don’t think you can assume people are going to just swap out one crop for another—that people with a traditionally corn-based diet are going to suddenly change their diets to rely on soy, or whatever.”
Overall, Price feels the models’ results foreshadow a future where food insecurity will be determined by whether a country’s crop productivity can keep pace with its population growth. If it can’t, which countries get the food they need and which don’t will be decided by the stability of global trade networks. “What comes out of this is that there’s a very strong dependence on human action and intervention,” says Price. “If we’re able to keep growing crops in new areas, and keep them fertilized and irrigated, then things don’t look that bad. But if it’s not possible to do that, then food insecurity starts to look like a real issue in the near future.”
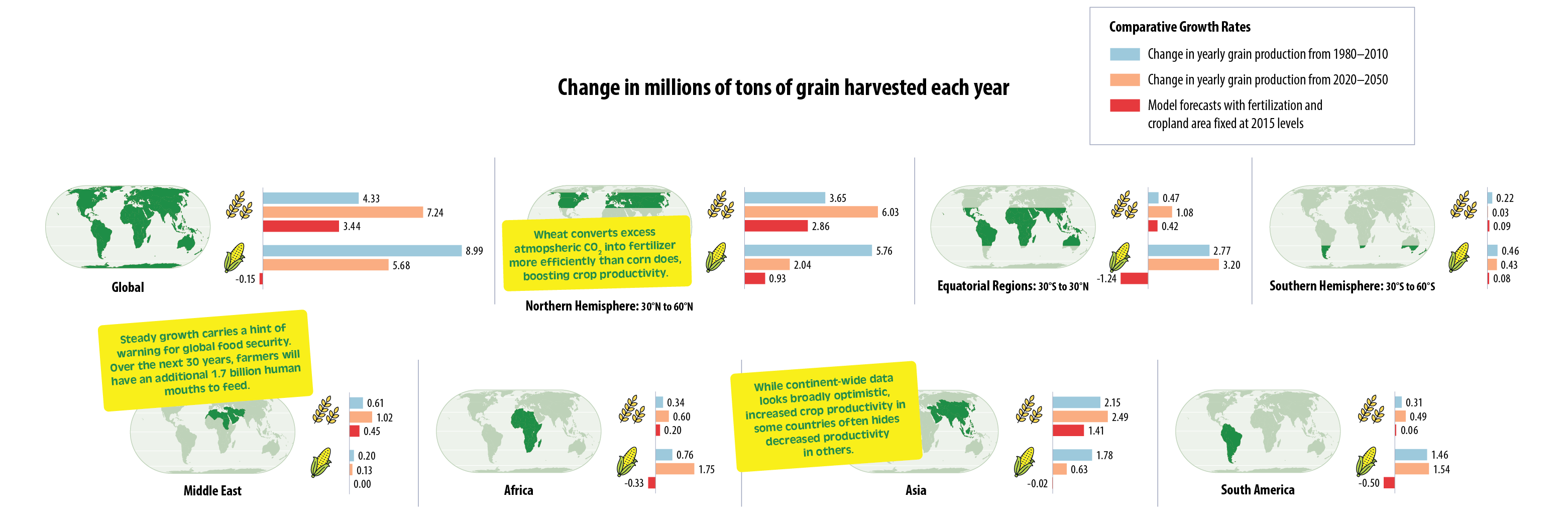
The upshot
Price is in his office, combing through some early data that showed global crop productivity climbing. He seems displeased. He can’t shake his belief that though they were modeling most of the key aspects of global climate, and therefore the biggest drivers of crop productivity, the pieces they were missing were skewing the results. The trends felt too…optimistic. “It’s hard to see how the factors our models aren’t yet considering could possibly make those projections more positive,” Price says.
He pulls up a slide on his computer that lists the drivers of crop productivity that his models don’t currently account for. Only one driver could benefit yields: drought-resistant crops, a field of genetics that could produce major returns on future crop yields. The other drivers on the list all have negative effects on yields. These include mid-season crop die-offs, pest and disease outbreaks, the accelerating loss and increasing nutrient deficiencies of topsoil, potential future limitations of the global fertilizer supply, and a heavy reliance on irrigation. To illustrate just how much these blind spots could swing results, Price points to the data from the runs he made after discovering the fertilizer error, the one that fixed fertilization, irrigation, and cropland area expansion at 2015 levels. Under this scenario, all major crops, in all parts of the world, responded the same way: Productivity decreased and yield projections flattened or began long, negative trajectories, a scenario that, given the world’s expected population growth, would result in historically high numbers of hungry people.
“It might be as simple as: what we do with land use is the most important thing,” says Price. “On its own, climate will have an impact that's negative in some areas and positive in others, but by far the biggest knobs we can turn are things that we at least nominally think we control: like how much land we devote to crops or how much fertilizer we apply.”
“It might be as simple as: what we do with land use is the most important thing.”
For Price, these results also underscored the importance of improving the models. He points to irrigation. Current models predict crop irrigation based on the crops’ needs alone. Plants are irrigated from a water supply that’s assumed to be unlimited. But, as anybody living in the American West knows only too well, that’s not how irrigation works. In May 2023, the federal government mandated that over the next three years, western states must collectively cut 3.3 million acre-feet, the volume of water contained in one acre when the water is one foot deep, from their share of Colorado River water, because the Southwest’s 20-year drought has very nearly sucked the source of most western irrigation dry. Fortunately, Lab researchers will be able to take advantage of efforts from other Department of Energy groups who are updating how the models represent irrigation. They are also developing a model with the very high resolution of one kilometer by one kilometer grid cells, which will allow predictions to be made at the scale of individual neighborhoods. “The better the models, the more confidence we’ll have in the results,” Price says.
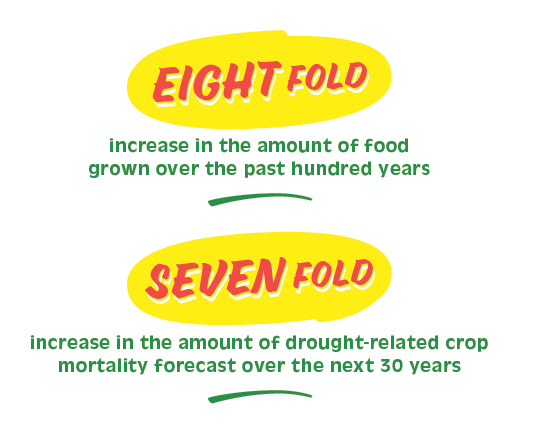
And the more confidence he’ll have in Earth system models’ ability to forecast the types of environmental changes that preceded the violence tearing Syria apart now. It’s a short step between a once well-fed people going hungry and those same people taking to the streets. The hope of Price, Pitts, and their team, is that a good prediction can help stop tragedy before it unfolds.