Determining the locations of geothermal hotspots—or places where the subsurface heat of the earth can be accessed—is something experts have been doing in various ways for years. From sifting through vast amounts of data to actually drilling deep into the ground to measure its temperature, identifying hotspots can take time, money, and effort. Now, scientists at Los Alamos are applying the techniques of machine learning (ML) to the discovery of hotspots—streamlining the process and aiding energy professionals in their search for explorable areas.
An ancient resource
Earth contains a lot of heat, some from the decay of radioactive minerals in the crust and some from the planet’s formation roughly 4.5 billion years ago. Mostly confined very deep underground, this heat is occasionally apparent at the ground surface. Obvious examples include natural hot springs, geysers like Old Faithful in Yellowstone National Park, and the magma inside volcanos. Hotspots like these are usually located along fault lines or near volcanoes.
Earth’s heat can be harnessed and used directly for a variety of purposes. Domestically it is used to heat homes, industrially it is used for hydrogen production, and commercially it is used in recreational spas and springs. But it is in electricity generation where the true potential of hotspots lies.
Out of every ten possible geothermal hotspots, two to five end up being unproductive.
Geothermal power plants use steam or water from underground that measures 300–700°F. From the 1970s to the 1990s Los Alamos scientists investigated hot dry geothermal power, in which the water was supplied externally, but so-called "hot dry rock" has still not achieved economic vitally. Underground water thus remains essential.
The water or steam is piped to the surface through wells drilled up to two miles deep into the ground. The steam or hot liquid is used to turn a turbine in the power plant, which then turns a generator, producing electricity.
There are three main types of geothermal power plants. First, dry steam plants use steam directly from the subsurface source to power generators. Second, flash steam plants convert high-pressure underground water into steam to turn the generator turbines. The steam is then cooled and condensed into water that is fed back to the deep subsurface reservoir. Lastly, binary cycle power plants transfer the heat from underground water to another liquid, which causes the second liquid to turn into steam to power the generators.
For more than a hundred years, geothermal energy has been an area of growing interest as scientists worldwide search for sources of renewable energy. The United States is a world leader in geothermal energy production, and New Mexico is sixth in the nation for geothermal potential, with hotspots largely located in the southwestern and north-central parts of the state.
However, finding hotspots by drilling wells is not as easy as poking holes in the ground. It is an expensive process that requires advanced technology, and that’s where Los Alamos scientists come in.
A modern method
“Even with all the expertise that exists in this field, it’s not well understood where or how to find geothermal hotspots,” explains recently retired Laboratory computational scientist Velimir Vesselinov, who led the project. “Most of the information and guidance regarding which geological settings hold high geothermal potential has been based on field findings—much like how the oil and gas industry used to rely heavily on field expertise to determine where to drill.” However, unlike the oil and gas industry, which has adopted a variety of technological advances to help find drilling locations, the development of novel exploration and drilling technologies for geothermal-energy research has largely stayed stagnant. Currently, geothermal reservoirs are individually located by analyzing and interpreting site-specific subsurface and geological characteristics.
“Typically, the experts will look at all the data and process it themselves,” says Bulbul Ahmmed, a postdoctoral researcher on the team. “They find the individual attributes, or variables in a dataset, that they believe are most important to defining the presence of geothermal reservoirs. Then, by accounting for the importance of each variable, they compute a composite score. Based on that score, they determine if a hotspot is likely to exist.”
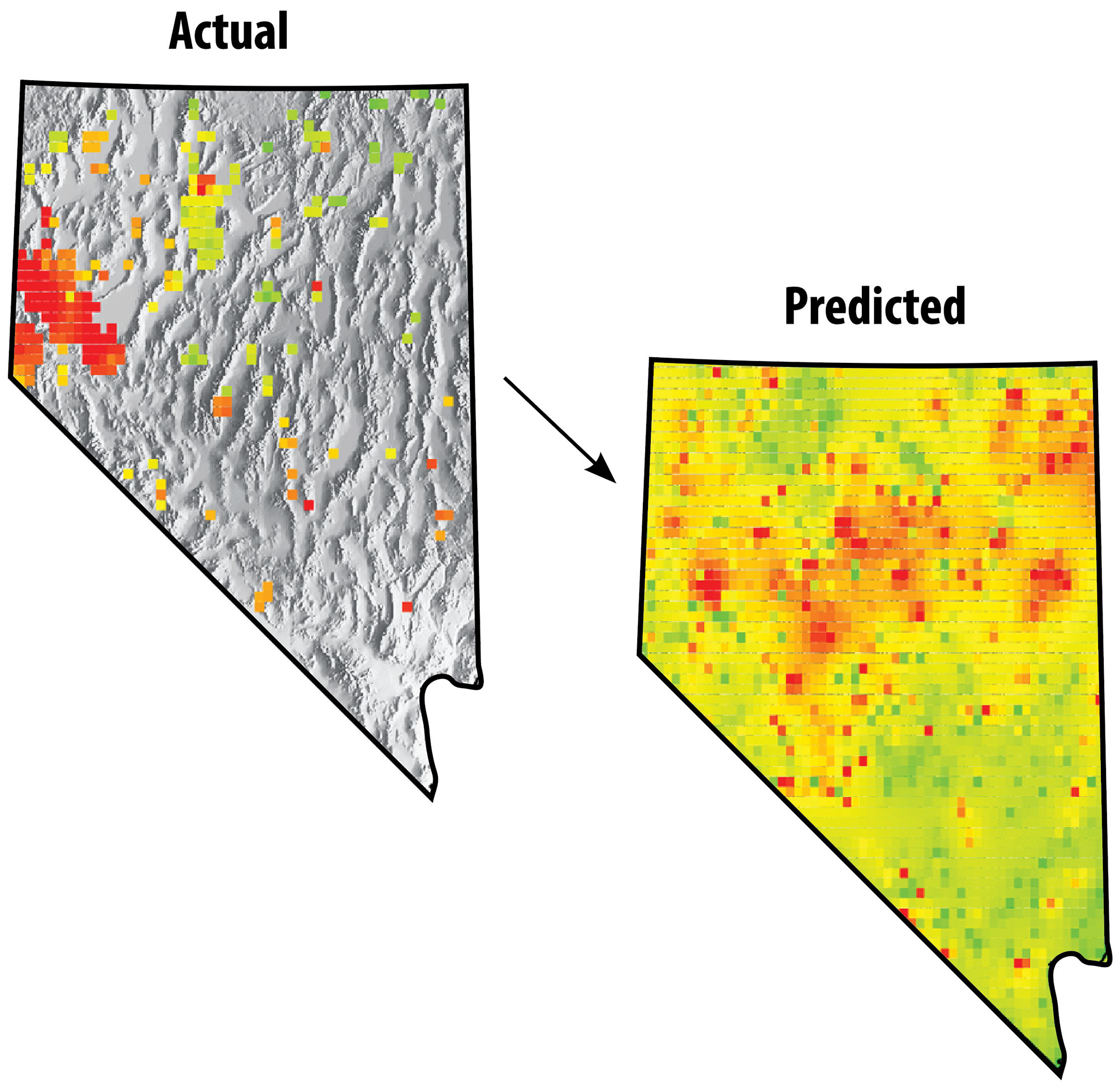
Even still, drilling a well and testing underground temperatures is the most reliable method for discovering and confirming a hotspot. In addition to being tedious, this process is costly and risky for companies. Out of every ten possible sources of geothermal energy, up to five end up being unproductive. After investing millions of dollars in a power plant to harvest that potential energy, if a reservoir fizzles out before a company can turn a profit, the cost deficit is a heavy one.
“Our ML method does two things: It removes human bias and it also analyzes every bit of information in a dataset simultaneously,” says Ahmmed. “That’s how we’re able to figure out what the interrelationships are between all the available data attributes, which traditional methods don’t allow.”
The Los Alamos team, in collaboration with several partner companies and the Department of Energy’s Geothermal Technologies Office, are applying ML to geothermal exploration and the discovery of reservoirs. This alleviates the need to rely on the inferences of experts in the field and, instead, brings a modern method for geothermal exploration to the industry.
“There is a lot of geothermal potential in the U.S., especially in the southwest,” says Vesselinov. “But it has not been sufficiently characterized. Exploration is expensive, and drilling is both very costly and difficult compared to oil and gas because of high temperatures and complex geology. What we’re trying to do is accelerate the exploration process.”
The Los Alamos project streamlines the process of finding areas that could provide abundant energy, by feeding geomorphological data into ML models. The models then process the data using algorithms and simplified codes to determine the locations of potential geothermal hotspots and to define which attributes are key to finding more prospective sites in the future.
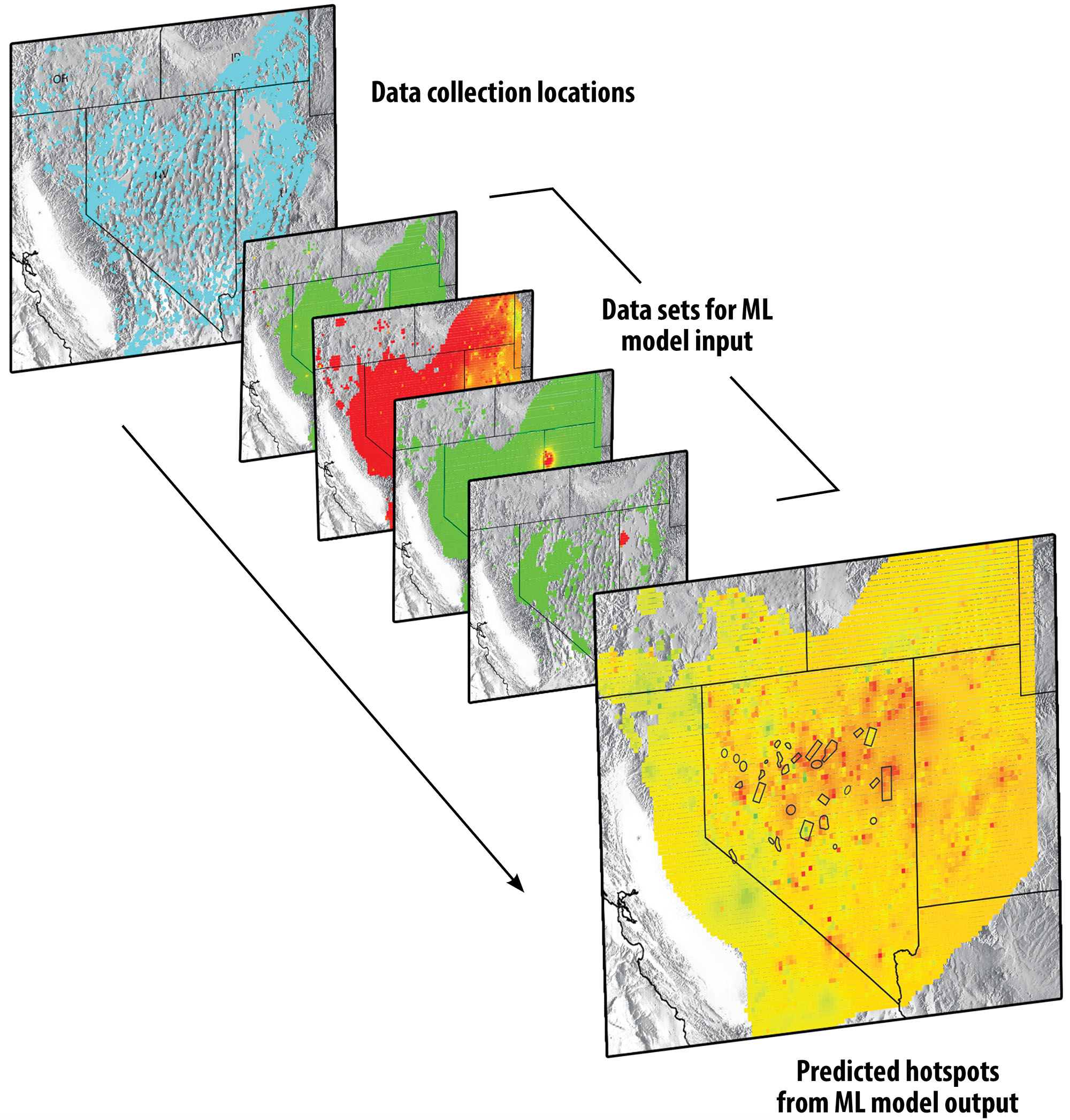
The team has developed fundamental ML algorithms to mine the available data and figure out what data are necessary to produce accurate results. ML is useful for geothermal ventures in that it acts much like a human brain—continuously taking new information that it is given and integrating it into its data memory and algorithms to improve its predictions of geothermal prospectivity. Additionally, the team’s software can analyze entire data sets all at once, instead of having to break them down and analyze each attribute independently.
An ML approach also removes human bias, as the technology examines all data points in a set, rather than picking which geological characteristics are more important than others in determining the location of hotspots.
“When humans do exploration research,” says Vesselinov, “they are more strategic and know what properties they need to look at for results, but they also might miss data that could inform their decisions because it’s impossible for a human to sift through the mountains of geological data. But a machine learning model can.”
The team tested its technology using unique data sets composed of information previously gathered throughout New Mexico, Utah, and Nevada. The findings were promising and resulted in the identification of several indicators of potential geothermal hotspots. In the Tularosa Basin in southwest New Mexico, for example, ML technology successfully singled out areas of interest by identifying geothermal signatures using key properties such as signals of heat flow, silica (often present in high concentrations in geothermal water), specific temperatures, and fault density.
“If ML tools are used in the right way for geothermal energy exploration, experts can mine much larger amounts of information than any human being could in a lifetime,” says Vesselinov.
While human experts are still needed to review the results, the ML methodology nonetheless acts as a magnifying glass helping to identify specific areas for further exploration.
The local connection
The team has recently applied its technology in a collaboration with a Navajo-, veteran-, and female-owned small business in New Mexico, called Tosidoh, LLC. Tosidoh is exploring how to best use a flowing hot water well, that was drilled by an oil exploration company in 1954 at Tohatchi Hot Springs.
After determining that the assessment of the well for geothermal energy was too complex of a task to tackle alone, Tosidoh joined the New Mexico Small Business Association to get technical support from Los Alamos researchers.
The scientists used their ML technology to combine data from a variety of preexisting documents about the well, and also to process and analyze new data gathered from the site. Then they further used ML to analyze geological, geophysical, and geochemical datasets to characterize the geothermal source and learn what subsurface processes were heating the water.
Machine learning acts as a magnifying glass, zooming in on where to explore.
“It was a very rewarding experience,” recalls Vesselinov. “Living in New Mexico, you always want to help the local economy and workforce. It was extremely exciting to work with Tosidoh. We also used the data from what we learned with them in our research because we didn’t initially have all the information from their geothermal site. Our joint project helped them, but they also helped us.”
The collaboration identified two aquifers beneath the well which could potentially support several projects, including a greenhouse-based farm, residential heating, a spa, and hydrogen production. Tosidoh is continuing to explore how best to use the well for sustainable applications and to provide local employment and revenue to the community and the larger Navajo Nation.
Driven by data
One of the most challenging aspects of ML technology is gathering enough data to develop, test, and verify ML models and their predictions. Once created, the models also have to be continually updated, integrating new relevant information as it becomes available. The Los Alamos team is reliant on data that has been collected over a 50-year time span, most of which wasn’t collected with the specific intention of using it for clean energy research. The data are limited in amount and can be low quality because they are sparse, incomplete, and at times, inaccurate. However, the team needs all the data it can get, so the scientists built the ML model to automatically pick out not only useful information but also suspected outliers or errors.
“Most machine learning methods are extremely data hungry,” says Vesselinov. “There is a classic example in ML of training a model to distinguish images of cats and dogs. The ML algorithm needs millions of images to learn what makes cats and dogs different. We don’t have millions of data points for prospective geothermal reservoirs. So, our model cannot be trained in the same way.” The team’s ML approach mines all the available data to extract latent features that are indicative of known geothermal hotspots; after that it looks for similar features at new sites. Based on the presence and strength of these features at new locations, the model produces estimates of geothermal prospectivity.
For example, in southwestern New Mexico, the team only had 44 locations to draw data from and only 18 data attributes informing geothermal conditions at each location. That’s less than 800 data points, compared to the millions of images in the dogs vs. cats example. Nevertheless, the team was able to identify different candidate locations based on distinctive inferred subsurface conditions.
While they have overcome the data-paucity challenge so far by meticulously pulling from all available sources, the team is also integrating new ways of collecting data. This will help “teach” their model to be more efficient and effective. This includes large diverse data sets such as satellite images and geophysics surveys, some of which are newly available thanks to recent advances in data acquisition and remote-sensing technologies.
“It’s been exciting to find new prospective resources with the little data we have,” says Ahmmed. “I think that’s what makes our ML method successful compared to other methods; we were able to get really important information from sparse data. However, now we are expanding our ML methods to mine larger and more diverse data sets coming from many different sources. It is like looking for a needle in a haystack, but our methods can do it very fast!”
The heat inside the earth is an ancient resource, some having been there since the planet formed. Only occasionally breaking the surface, it has mostly stayed hidden deep below. Now, thanks to modern technology like ML, scientists can find and put to use the heat beneath our feet.